LLMs, enterprise AI strategy, and the future of the TMS | Q&A with Sara Basile
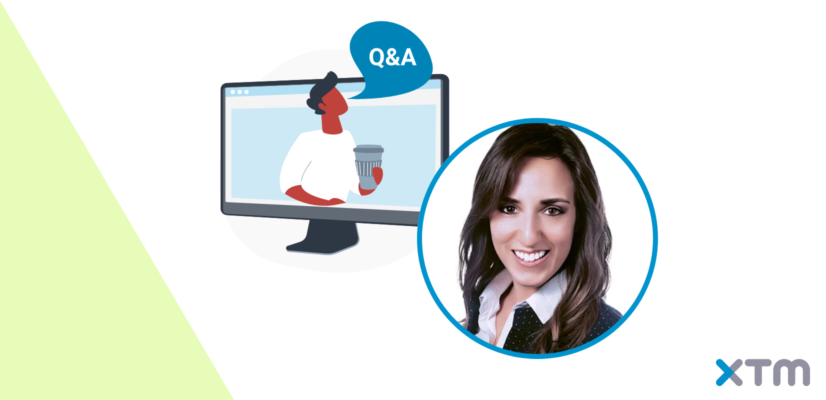
The conversation around AI in the localization industry has shifted from talking about what it can do to understanding what AI strategy localization-technology providers have in place and how their customers can benefit from it moving forward.
In this conversation with XTM Product Director Sara Basile, she talks about XTM’s present and future AI strategy, how it plans on incorporating LLMs, and what the future holds for the TMS and why it’s still going to be very much relevant.
Q: Can you tell us a bit more about what we can expect from XTM in the AI sphere this 2024?
A: We’ve got some very exciting AI features in the pipeline! In the second half of the year, we are going to complete our Beta program including Translation Quality Evaluation, GPT integration with SmartContext, and QA checks for gender-biased and inappropriate language. I’d like to say a big thanks to all our beta customers and their engagement throughout the program. Thanks to their feedback, we’re already introducing significant improvements to all these features, which will allow us to get them ready to go live in the next release.
Anything else lined up?
Of course! Next up in the pipeline we have features on automatic post-editing and prompt customization on the fly. Smart, AI-driven workflows are also going to be a big theme at XTM. As scalability and customization are at the heart of what we do, we are also looking forward to offering our customers additional LLM options and easier orchestration functionality – watch the space for additional announcements later this year.
People want to hear about real use cases for AI tools and what challenges they help with. What problems are we helping customers solve with our AI technology?
GenAI is undoubtedly raising the bar when it comes to the expectations for global business growth. Now, companies around the world feel empowered to create multilingual content at scale, reducing time to market and internal costs. However, when it comes to adopting GenAI at scale, there is enough market research out there proving that the biggest concern for enterprises trying to adopt GenAI at scale is Quality.
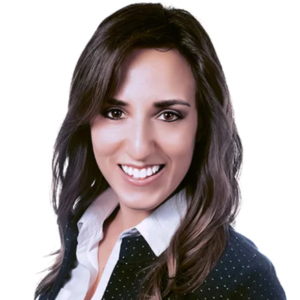

LLMs have proven to be great at digesting context to improve their output. So where can that context be taken from if not from the TMS?”
Sara Basile
Product Director
Is that the feedback that you get from customers?
Yes, and it’s understandable. We often hear that GenAI still feels a bit like a black box – for example, why did the LLM produce this translation, or why did it produce this score? According to the recent IBM Global AI Adoption Index Report, IT professionals are largely in agreement that consumers are more likely to choose services from companies with transparent and ethical AI practices and say being able to explain how their AI reached a decision is important to their business.
So how do you “explain” AI?
The concept of Explainable AI is very important to us. For example, when it comes to the translation quality evaluation QA check, we are planning to introduce ways of guiding our users through the decisions made by the LLM and stay in control, so that it feels less like a black box and more like a tool that behaves according to what the user needs. XTM has always been about empowering users to decide how to leverage the tool to achieve their business goals.
What examples of this do you have?
For example, our AI quality assurance checks about inappropriate and non-inclusive language, as well as the translation quality evaluation functionality are meant to give our customers the right tools to stay in control when required. Both functionalities now in Beta run as QA checks for now and they run on any content, both machine or human-translated. This means that not just a post-editor or proofreader can leverage QA to easily pinpoint the most critical issues at a glance, but these checks can also run on the source before sending the content off for translation.
There are questions about how AI can (or cannot) handle context in its generated content. How do you overcome this?
Context is very important when generating content in any language. LLMs have proven to be great at digesting context to improve their output. So where can that context be taken from if not from the TMS where all translation memories and terminology are stored?
With our XTM AI SmartContext feature, also currently in Beta, we want to give our users exactly this possibility of creating content at high speed (through an LLM like GPT) and at the same time without having to compromise on quality and brand consistency – without the human in the loop or with hybrid workflows paired with advanced, AI-driven QA checks.
And what are you doing to appease any concerns customers may have about data security and quality control with AI?
The value that has always driven XTM as an organization for more than 20 years is that the customer comes first, and the same applies to their data. We started to release our first AI features almost a decade ago with the TM aligner. Then in 2020 came the automatic placement of inline tags, which was then directly followed by additional improvements like BTE, and many more functionalities.
With these features, we have committed to treating our customer data with the highest security and confidentiality standards. But now, with GenAI, we realize that it’s becoming essential to officially share our AI principles.
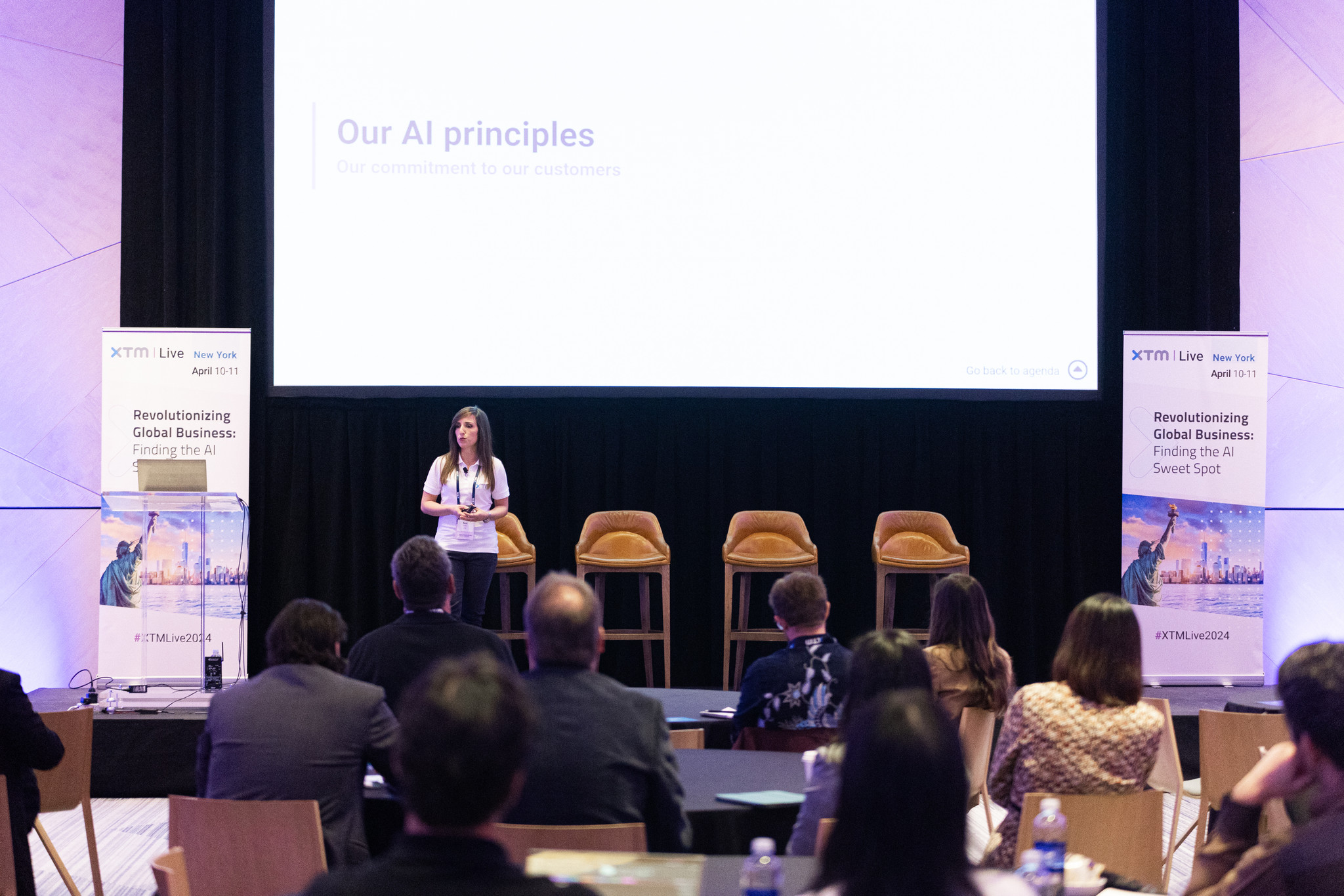
Sara Basile at XTM Live 2024 in New York City.
You’ll soon be on the panel of a webinar on how to reduce post-editing time with LLMs in XTM. Can you tell us a bit more about what potential benefits LLMs offer to post-editing workflows?
In many situations, post-editing proved to be a great use case to start “small” and experiment with LLM quality before using it for translation from scratch. LLMs are great at producing output based on context, so by instructing the LLM to start from a text and improve it based on defined criteria, we were able to witness great results.
Automatic post-editing has the potential to dramatically reduce time to market by increasing linguist productivity, as it can improve the text by referencing style guides and glossaries on the fly or following additional instructions through prompt customization. In this way, the LLM becomes a smart assistant that can be flexibly used at any point during the workflow.
What do you expect will be the role of the TMS after the emergence of proprietary LLMs? Will it be phased out or is it still going to be a key tool in localization?
GenAI has led to an explosion of content. It requires more attention to quality with faster processing and time to market. As was the case with the rise of NMT, the concept of a TMS is just evolving, just as the role of localization professionals is also evolving.GenAI comes with considerable Scalability, Quality, and Transparency challenges for Enterprises. In the context of multilingual content creation, it becomes imperative to introduce fast and reliable quality-assurance processes that avoid the spread of mistakes leading to brand reputation damage. This is only possible with scalable QA processes that empower customers to leverage both AI and humans in combination and to the extent that makes the most sense to them based on their requirements.
So what will be the role of tech providers in a company’s AI deployment?
Companies looking at implementing mature, AI-driven workflows at scale require reliable technology partners and a tool that takes over the “heavy lifting” of file processing, workflow orchestration, asset management, and quality assurance for brand consistency. This is paired with interoperability and connectivity to CMSs, repositories, and several LLMs that are orchestrated to provide the best output based on different criteria. Experimenting with LLMs or introducing small cases is a great starting point, but to create real business value, it’s essential to have a system that helps you scale, and that’s where the value of tech companies will lie.
Would you like to find out more about XTM’s AI tools and how they can help your business become more efficient?
Related articles
Looking for more content? Check out these suggestions.