About the company
Ariel Corporation is the quintessential American entrepreneurial company. It was founded in a basement in 1966, and more than five decades later the business has grown into the largest manufacturer of separable reciprocating gas compressors worldwide, while still retaining its ethos and remaining family run.
Today, Ariel employs over 1,500 staff in multiple cities across Ohio, with its compressors used to extract, process, transport, store and distribute natural gas.
Ariel’s compressors are used in over 100 countries worldwide. In order to provide top-notch service to its global customers, Ariel translates its content from English into Spanish, Chinese and Russian.
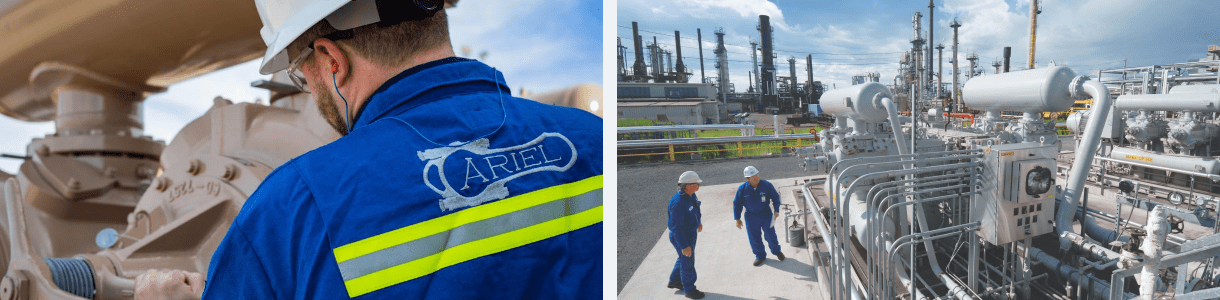
The challenge
Ariel works in partnership with Argos Multilingual and freelance translators to translate its English website, support portal, video, technical documentation and online learning course content into Spanish, Chinese and Russian. The team uses the Adobe Experience Manager content management system, which feeds their content into and out of XTM for translation via a connector. Because this process is completely automated, the localization team can spend minimal time managing their translation operations. It was an excellent setup. However, Ariel needed to increase translation output while reducing overall localization spend, so they decided to explore the possibility of making this process even more efficient.
Ariel’s key localization challenges:
Reduced post-editing time
Increase translation output
Minimize localization spend
The solution
In 2019 Ariel started to investigate how they could use machine translation to reduce human translation and review time and, by extension, cut costs. They teamed up with SYSTRAN MT, aiming to use machine translation (MT) to translate all of their second- and third-level website content.
Ariel implemented SYSTRAN MT in 2020 and subsequently integrated with XTM and AEM via connectors to make the whole process as streamlined as possible.
Ariel’s integrated MT workflow:
In this new, enhanced workflow, the Adobe Experience Manager (AEM) content management system sends the original document to XTM for processing. The translation memory is checked for matches, then the document is sent to SYSTRAN for machine translation. MT post-editing is performed by Ariel’s translators, before being reviewed by its regional officers. They run a quality assurance check, then the final translation is automatically sent back to AEM for publishing
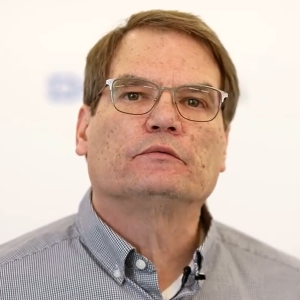
By using XTM and SYSTRAN, we’ve been able to find extra value in our language assets.”
Ronald Egle
Content Systems Administrator, Ariel Corporation
Having reaped the rewards of this approach, Ariel started wondering whether they could push the boat out. Could they teach SYSTRAN to improve MT output even further? In other words, could translators’ corrections teach the MT engine to produce higher quality translation? And in so doing, reduce human translation and editing time and overall spend? It was a good idea. So good in fact that they weren’t the only ones to think of it… It turned out XTM was already working with SYSTRAN on developing just such a system.
In April 2021, XTM released version 12.7 with its ground-breaking neural fuzzy adaptation (NFA). In computer assisted translation (CAT) tools, a fuzzy match refers to a similar yet nonidentical segment, or string of text, in a translation memory. Say you’ve translated a very similar sentence before, but with one or two words different. By using fuzzy matches, the CAT tool will suggest your previous translation, with a score below 100% to indicate the discrepancies. Normally, you’d input the translation suggestion, then manually make the one or two required changes. But NFA turns this process on its head.
NFA uses artificial intelligence and data from your previous translations to analyze and ‘repair’ a fuzzy match, transforming it into a full match. So, in the above scenario, it would replace those words automatically, meaning that humans don’t need to intervene at all.
The outcome
The continual enhancements to XTM, and in particular, the addition of SYSTRAN MT with neural fuzzy adaptation, have allowed Ariel to reduce the amount of time and effort humans need to spend translating and reviewing their content. Consequently, Ariel has been able to get more from their translators and language assets, while reducing overall localization spend.
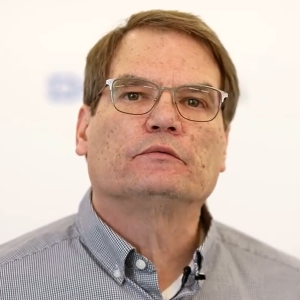
We had a phenomenal setup with our content management system feeding automatically into and out of XTM. Adding SYSTRAN MT to the process made it even better. Then with the release of XTM 12.7 and neural fuzzy adaptation, we found gold. Now we’re able to harness the very best of both machine translation and human editing for outstanding cost efficiency.”
Ronald Egle
Content Systems Administrator, Ariel Corporation
In 2020, before the release of NFA, machine translation accounted for 17% of Ariel’s overall translation. NFA’s implementation in 2021 reduced the company’s human translation efforts by an impressive 31%, with the quality of machine translation improving by 100%.
With broader integration into XTM, NFA has the potential to reduce meticulous editing even further by including 85-99% fuzzy matches. By extension, NFA-translated segments have the potential to increase MT translation by 129%.
In addition, having this vastly improved MT engine provides Ariel’s translators with a second, high-quality reference source when translating new segments. While this benefit is hard to measure, it undoubtedly reduces post-editing time.
As Ariel looks to the future, the company is interested to see how they can drive down translation and review costs even lower by focusing on their content creation processes. For instance, the localization/content team is in the process of working with their writers to structure their content using predictable patterns and language. This will enable machine translation to be used to an even greater extent, making localization smoother and more efficient. In addition, the localization team is interested in how they can achieve even greater levels of automation by incorporating AI into all aspects of their translation processes.