1. Let Weighted Token Levenshtein (WTL) boost your bottom line
As mentioned above, being able to retrieve matches from translation memory tools as quickly as possible is a crucial part of effective localization. At XTM, we originally adopted the industry-standard methodology for leveraging previously translated translation memory content, known as Q-gram, which examines phrases and searches for corresponding character patterns. However, there is a problem with Q-gram; it was unable to take syntax into account. For us, this meant that the maximum leverage you could get was not met, which was not good enough for us. We wanted a translation memory software mechanism that would be smarter, and find more matches stored in the translation memory without adding more work for the user.
This is why we created our own proprietary TM-leveraging algorithm, which we named Weighted Token Levenshtein (WTL). This algorithm generates fuzzy matches by considering and recognizing the syntax of the segments in the localization project, allowing us to retrieve more matches than any other TMS’s translation memory software would allow.
WTL is able to spot matches that normally wouldn’t be recognized, such as sentences that differ by word order, like “To find out more about XTM Cloud’s new features, check our website.” and “Check our website to find out more about XTM Cloud’s new features.” With Q-gram, linguists would have to rewrite the whole segment, whereas WTL can spot that these sentences are nearly identical and will provide a 75% match. Similarly for segments like “Visit Paris” and “Visit London”. In theory, only half of this segment (the word “visit”) would get recognized in the TM. This equates to a 50% match, which is too low to be picked up and leveraged by the CAT tool. WTL, on the other hand, can recognize that “Paris” and “London” are proper nouns, and will apply this logic when calculating what kind of match it is. Translation memory is able to recognize that these two segments are actually almost identical, retrieving a 92% match as shown in the below video:
With WTL, the Translation Memory is fully utilized, ensuring that linguists can work efficiently and not waste time localizing segments that have already been translated—time and cost that you will not have to invest in.
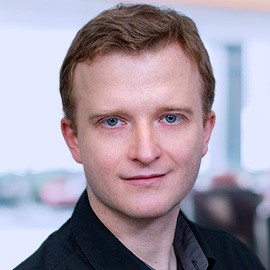
XTM concentrates on providing robust, efficient, and innovative AI solutions by combining in-house development with services delivered by external AI providers. XTM’s unique developments, e.g. Inter-language Vector Space, are providing high-precision text analysis in various languages. External integrations, e.g. Systran NFA or planned OpenAI GPT leverage the latest achievements in deep learning to achieve amazing results.”
Dr. Rafał Jaworski
Linguistic AI Expert
2. Save 50 hours with the Translation Memory aligner tool
When localization teams are trying to match source and target segments, a process called “translation memory alignment” is required. This can occur if your team did not use a translation memory tool during the localization process or if you are migrating to a new TMS tool, and translation memories and segments were not included in the migration process. That new TM could take months to build from the ground up—XTM Cloud can accomplish this in minutes.
XTM Cloud’s translation memory software will analyze previously translated source and target segments and generate translation memories based on them. It will also enable you to expand your existing TM by uploading files that you had not previously uploaded. This process is not always as straightforward as it may appear. There could be many differences, such as single sentences in English that need to be translated into two to three sentences in the target language, or vice versa. The aligner will detect these without any user intervention.
To perform TM alignments with legacy translation memory tools, you would have had to manually draw a line between the source and target segments, which was extremely time-consuming. Now, although automation has been added, with most translation memory tools you would still need to manually verify that each segment has been properly aligned and correct any mistakes. If you need to create translation memories from large documents with hundreds of thousands of segments, this becomes a real problem. For instance, with a manual alignment speed of 15 segments per minute, preparing a 50,000-segment translation memory would take over 3,000 minutes, or more than 50 hours. This is the type of volume that our TM aligner can handle in a matter of minutes.
3. Find the perfect match with SYSTRAN AI-enhanced TM and GPT
Translation memory (TM) and Machine Translation (MT) engines previously only coexisted without ever really working in tandem, with the TM primarily used for “training” the MT engine. Now, Neural Machine Translation (NMT) engines effectively train themselves on the fly using the translation memory in a self-sustaining, interactive process, paving the way for high-quality translation at scale. Here’s how it works.
AI-enhanced Translation Memory (TM) combines cutting-edge TM and NMT functionalities to create something greater than the sum of its parts. It enables the machine translation engine to deliver full matches using fuzzy matches. When a sentence is already partially translated, the Neural Machine Translation (NMT) engine can use existing matches to fill in the gaps and deliver a complete translation, or even adjust the fuzzy match if needed. The segment is completed instantly, with little or no rework required.
This is particularly useful when one word could have a variety of different meanings, such as “glasses”: it could be referring to wine glasses or prescription glasses, for instance. In this case, AI-enhanced Translation Management can find the correct translation based on previously localized content. The segment can be localized in full, requiring no rework from linguists. The below video illustrates this example:
Ariel Corporation, the largest manufacturer of separable reciprocating gas compressors worldwide, used this functionality to localize its content from English into Spanish, Chinese, and Russian. The results speak for themselves, as the company reported that since implementing the AI-enhanced translation memory technology, they have been able to:
- Improve machine translation quality by 100%
- Increase their translation output
- Reduce human translation by 31%
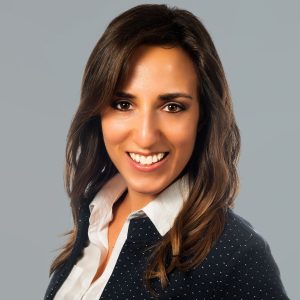
Like humans, feedback is key to improving AI’s performance as well. Translation memories are a goldmine of information related to your company brand voice in multiple languages, so there’s no better resource than a Translation Manager to give feedback to AI on how to improve. And even better, when this happens on the fly without any manual intervention. This is what we have achieved in partnership with SYSTRAN with our AI-enhanced Translation Management feature, and this is what we are soon going to achieve with our cutting-edge integration with Open AI’s GPT technology.
I look forward to seeing our customers reaping the benefits of Large Language Models straight from their enterprise TMS of choice.”
Sara Basile
Product Director
Available Now: XTM AI Smart Context
XTM AI SmartContext leverages the power of OpenAI GPT-4.o mini, to automatically generate high-quality translations based on fuzzy matches. This cutting-edge feature ensures consistency and speed, empowering you to maintain your brand’s voice effortlessly across all content. By analyzing your translation memory and considering style, and tone, XTM AI SmartContext delivers on-brand translations with minimal effort, reducing the time and resources spent on post-editing. Experience the reassurance of flawless translations that reflect your brand’s unique tone with minimal effort, making your localization process smoother and more efficient.